Imagine a system that can predict risks in your project before they even happen, much like how algorithms can predict your next favorite song. AI-driven risk analysis does just that—transforming risk management into a predictive, data-driven process.
Did you know that AI can process vast amounts of data in milliseconds, identifying patterns invisible to the human eye? In fact, AI is used to detect space anomalies and even map the human genome!
Now, it's revolutionizing how organizations foresee and mitigate risks, offering faster, more accurate insights.
In this article, we will delve into:
- Discover how AI is transforming project risk assessment
- Learn to implement smart risk assessment in your projects
- Explore real-world success stories of AI-driven risk analysis
- Compare AI and traditional methods for better risk management
Get ready to explore how this cutting-edge technology is reshaping the future of project risk management in ways we never thought possible.
The Revolutionary Impact of AI on Project Risk Assessment
In the rapidly evolving landscape of project management, AI-driven risk analysis has emerged as a game-changing approach, transforming how organizations identify, assess, and mitigate potential risks.
This innovative methodology is not just an incremental improvement; it's a paradigm shift that's reshaping the very foundation of project risk assessment.
Redefining Risk Analysis with AI
At its core, AI-driven risk analysis leverages the power of machine learning and advanced algorithms to process vast amounts of data and generate insights that were previously unattainable.
This approach goes beyond traditional statistical models, employing sophisticated techniques that can:
- Identify subtle patterns in complex datasets
- Predict potential risks with remarkable accuracy
- Learn and adapt to new information in real-time
The fundamental concept behind AI-enhanced risk prediction is the ability to augment human decision-making with data-driven insights.
By combining the analytical power of AI with human expertise, organizations can achieve a more comprehensive and nuanced understanding of project risks.

Key Advantages Over Traditional Methods
AI-driven risk analysis offers several significant advantages over conventional risk assessment techniques:
- Enhanced Accuracy: AI models can process and analyze vast amounts of data, considering a wider range of variables than humanly possible. This leads to more precise risk predictions and fewer overlooked threats.
- Real-Time Analysis: Unlike traditional methods that often rely on periodic assessments, AI systems can continuously monitor project data, providing up-to-the-minute risk insights.
- Predictive Capabilities: AI algorithms can identify subtle patterns and correlations that humans might miss, enabling proactive risk management rather than reactive responses.
- Scalability: AI-driven systems can easily handle projects of any size or complexity, from small local initiatives to global, multi-faceted programs.
- Objective Assessment: By relying on data rather than gut feelings or personal biases, AI-driven analysis offers a more impartial evaluation of potential risks.
- Customization: Machine learning models can be tailored to specific industries or project types, providing highly relevant and contextualized risk assessments.
As AI technology continues to advance, we can expect even more sophisticated and powerful risk analysis capabilities. The future of project risk assessment is here, and it's being driven by the incredible potential of artificial intelligence, as exemplified by these pioneering companies.
Implementation of Intelligent Risk Assessment in Projects
Integrating AI-enhanced risk management into project workflows can significantly improve outcomes. However, successful implementation requires a strategic approach.
Let's explore the key steps, challenges, and best practices for bringing intelligent risk assessment to your projects.
Roadmap to Integration
- Evaluate and Plan: Begin by assessing your current risk management processes. Identify specific areas where AI can add the most value, such as predicting cost overruns or scheduling conflicts.
- Prepare Data: The success of AI systems heavily depends on data quality. Audit your data sources, ensuring they're comprehensive, accurate, and relevant. Implement data cleaning and normalization procedures as needed.
- Select and Pilot: Choose an AI-powered risk analysis tool that fits your organizational needs. Consider factors like scalability and integration capabilities. Start with a small-scale pilot project to test the chosen tool and gather feedback.
- Train and Deploy: Provide comprehensive training for your team. Develop user guides and establish a support system. Gradually roll out the AI risk analysis tool across all relevant projects.
- Refine and Improve: Regularly review and refine the AI models based on new data and outcomes. Stay updated with the latest advancements in AI risk analysis technology.
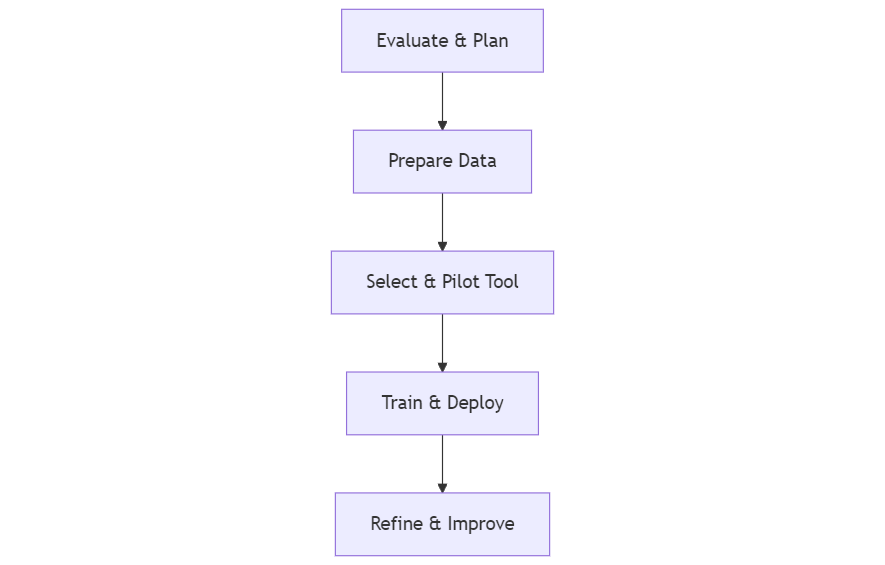
Navigating Adoption Challenges
Implementing new technology often comes with hurdles. Here are common challenges and strategies to overcome them:
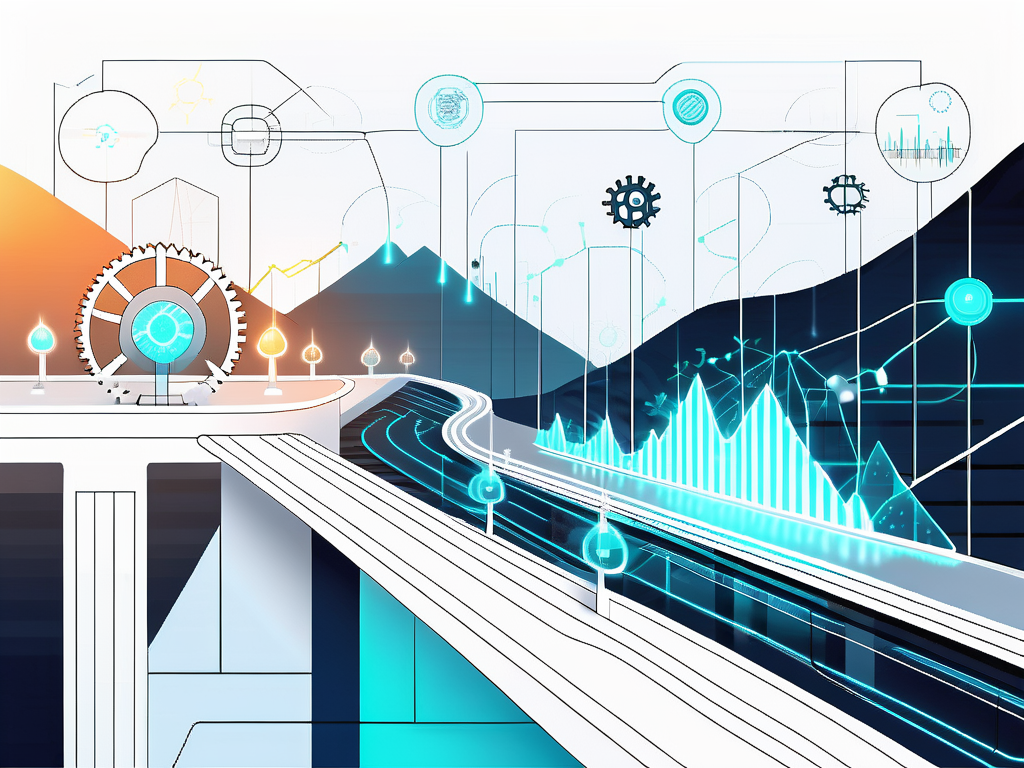
- Data Quality Issues: Invest in robust data collection and cleansing processes. Consider external data sources to enrich your datasets.
- Resistance to Change: Emphasize the benefits of AI-driven risk analysis through clear communication and demonstrations. Involve key stakeholders early in the implementation process.
- Integration Complexities: Choose AI risk analysis tools with strong integration capabilities. Work closely with IT teams to ensure seamless connectivity with existing systems.
- Skill Gap: Provide comprehensive training programs. Consider partnering with AI consultants or hiring specialized talent to bridge knowledge gaps.
Best Practices for Maximum Impact
To fully harness the power of intelligent risk assessment in your projects, consider these best practices:
- Foster a Data-Driven Culture: Encourage teams to base decisions on AI-generated insights rather than gut feelings. Regularly share success stories and tangible benefits of AI-driven risk management.
- Maintain Human Oversight: Use AI as a tool to augment human decision-making, not replace it. Establish clear protocols for when human intervention is necessary.
- Ensure Model Transparency: Opt for AI systems that provide explainable results. Regularly audit AI models to understand their decision-making processes.
- Prioritize Data Security: Implement robust data protection measures to safeguard sensitive project information. Adhere to relevant data privacy regulations and industry standards.
- Embrace Continuous Learning: Stay updated with the latest developments in AI risk analysis. Encourage ongoing education and skill development among team members.
By following these guidelines, organizations can successfully implement AI-enhanced risk management systems. The result is more resilient and successful projects that drive business growth and innovation.
Real-World Examples of Successful Implementation
The transformative power of Intelligent risk assessment is already evident across various industries, with several well-known companies leading the charge:
Autodesk: Revolutionizing Construction Risk Management
Autodesk, a leader in design and engineering software, has integrated AI-enhanced risk prediction into its Construction Cloud platform. This system has been adopted by major construction firms worldwide, including Skanska and BAM Construct UK, with impressive results:
- 40% reduction in safety incidents
- 25% decrease in project delays
- 20% cost savings due to improved resource allocation
The AI system analyzes historical project data, weather patterns, and real-time sensor information to predict and mitigate risks proactively.
For instance, BAM Construct UK used the platform to identify potential clashes in building designs before construction began, saving millions in potential rework costs.
JPMorgan Chase: AI-Powered Contract Intelligence
JPMorgan Chase, one of the world's largest banks, developed an AI system called COIN (Contract Intelligence) for risk analysis and contract review. The impact has been substantial:
- 360,000 hours of lawyer time saved annually on contract review
- 99% reduction in loan-servicing errors
- Significant improvement in identifying potential regulatory issues
COIN analyzes complex legal documents and extracts important data points, allowing for more comprehensive risk assessments. This system has revolutionized JPMorgan's approach to contract analysis and risk management.
Roche: Enhancing Healthcare with AI Risk Analysis
Roche, a global healthcare company, has implemented AI-augmented risk identification in its NAVIFY platform for personalized healthcare solutions. The system has been particularly impactful in oncology:
- 30% reduction in time to treatment for cancer patients
- 40% improvement in identifying potential drug interactions
- 25% increase in clinical trial matching efficiency
NAVIFY analyzes vast amounts of medical literature, clinical trial data, and patient information to identify potential risks and optimize treatment plans.
For example, it helped oncologists at the Mayo Clinic make more informed decisions about patient care by providing comprehensive risk assessments based on the latest clinical data.
Google: AI-Optimized Data Center Management
Google has employed AI-enabled risk forecasting, developed by its DeepMind division, to optimize data center cooling systems. The results have been remarkable:
- 40% reduction in energy used for cooling
- Significant decrease in the risk of data center outages
- 15% improvement in overall power usage effectiveness (PUE)
The AI system analyzes thousands of data points from sensors to predict temperature and pressure changes, adjusting cooling systems in real time to minimize energy usage while maintaining optimal operating conditions.
Amazon: AI-Driven Supply Chain Risk Management
Amazon has implemented a sophisticated predictive risk modeling system to manage its vast global supply chain:
- 35% reduction in supply chain disruptions
- 20% improvement in inventory forecasting accuracy
- Significant cost savings through optimized logistics
The system analyzes data from multiple sources, including weather forecasts, traffic patterns, and supplier performance metrics, to predict and mitigate potential supply chain risks. This has been crucial in maintaining Amazon's promise of fast and reliable delivery, especially during high-demand periods like Prime Day and the holiday season.
These real-world examples from industry leaders demonstrate the transformative potential of AI-driven risk analysis across diverse sectors.
By embracing this innovative approach, organizations can not only mitigate risks more effectively but also unlock new opportunities for growth and success.
AI-Driven vs. Traditional Risk Analysis: A New Era of Project Risk Management
In the ever-evolving landscape of project management, risk analysis stands as a crucial pillar for success. As we venture further into the digital age, a new contender has emerged to challenge traditional risk analysis methods: AI-driven risk analysis.
Let's explore how these two approaches compare, focusing on their accuracy, speed, and scope.
Accuracy: Precision in Prediction
Traditional Methods: Rely heavily on historical data and expert judgment. While valuable, these methods can be subject to human biases and limitations in processing vast amounts of data.
AI-Driven Approach: Leverages machine learning algorithms to analyze enormous datasets, identifying subtle patterns and correlations that humans might miss. This leads to more accurate risk predictions, especially for complex projects with multiple variables.
Speed: Racing Against Time
Traditional Methods: Often involve time-consuming manual processes, including data collection, analysis, and report generation. Updates to risk assessments can be infrequent due to resource constraints.
AI-Driven Approach: Offers real-time risk analysis, continuously processing new data as it becomes available. This allows for immediate updates to risk profiles and swift responses to emerging threats or opportunities.
Scope: Broadening the Horizon
Traditional Methods: Typically focus on known risks and may struggle to identify emerging or unforeseen risks. The scope is often limited by the expertise and experience of the risk assessment team.
AI-Driven Approach: Capable of analyzing a wider range of variables and data sources, including unstructured data like social media trends or news articles. This broader scope allows for the identification of novel risks and opportunities that might be overlooked by traditional methods.
Visual Comparison

While traditional risk analysis methods have served us well for decades, the advent of AI-driven approaches marks a significant leap forward in our ability to manage project risks.
The enhanced accuracy, speed, and scope offered by AI enable project managers to make more informed decisions, respond faster to changes, and identify a broader range of potential issues.
However, it's important to note that AI-driven risk analysis is not about replacing human expertise, but rather augmenting it. The most effective risk management strategies often combine the analytical power of AI with the nuanced understanding and experience of human experts.
Embrace The Future Of Risk Management With AI
AI-driven risk assessment is transforming how projects are managed, offering unparalleled accuracy, speed, and scalability.
By integrating AI into your risk management processes, you can identify threats in real-time, predict potential issues before they arise, and make data-driven decisions that keep your projects on track.
Combining the power of AI with human expertise leads to better project outcomes and ensures that risks are managed proactively, not reactively.
Ready to elevate your risk management strategy? Unlock the full potential of your projects with AI-powered project management software. Streamline your workflows, mitigate risks effectively, and deliver flawless results.