Did you know that unplanned downtime costs businesses up to $50 billion annually? Even more surprising—over 80% of industrial failures happen randomly, without warning. This is where AI for Predictive Maintenance steps in, turning guesswork into data-driven precision.
Imagine machines that predict their own failures before they happen! In this guide, we’ll explore:
- Unleash the power of AI to transform maintenance
- Follow this proven AI implementation roadmap
- Learn from real success stories in AI maintenance
Game-Changing Technologies Powering AI-Driven Predictive Maintenance
The success of AI-driven predictive maintenance depends on a combination of cutting-edge technologies that enable machines to detect anomalies, predict failures, and optimize maintenance schedules with incredible precision.
Below, we explore the key innovations that make predictive maintenance smarter, faster, and more reliable.
The Power of Machine Learning in Maintenance
At the heart of modern predictive maintenance lies three distinct types of machine learning approaches, each serving unique purposes in maintenance prediction:

Supervised Learning acts like an experienced maintenance engineer, learning from historical data where we know both the equipment conditions and the eventual outcomes. This approach excels at predicting specific failure types based on patterns it has seen before.
For example, it can learn that certain vibration patterns in a motor typically lead to bearing failure within 48 hours.
Unsupervised Learning works more like a detective, discovering hidden patterns in maintenance data without needing historical failure examples. This makes it particularly valuable for identifying unusual equipment behavior that might indicate emerging problems, even if we've never seen that specific issue before.
Reinforcement Learning operates like an adaptive maintenance scheduler, continuously improving its decision-making based on the outcomes of previous maintenance actions. This approach is particularly powerful for optimizing maintenance timing and resource allocation.
Deep Learning and Neural Networks: The Brain Behind Predictions
Deep Learning neural networks have revolutionized predictive maintenance by processing vast amounts of complex sensor data in ways that mimic human brain function. These sophisticated networks excel at:
- Pattern Recognition: Identifying subtle equipment deterioration patterns that might be invisible to human observers
- Multi-sensor Integration: Combining data from various sensors to form a comprehensive understanding of equipment health
- Predictive Accuracy: Achieving unprecedented levels of accuracy in failure prediction through deep learning architectures

Time Series Analysis: Understanding Equipment Behavior Over Time
Modern predictive maintenance relies heavily on advanced time series analysis to understand how equipment performance changes over time. This technology enables:
- Real-time Anomaly Detection: Instantly identifying when equipment behavior deviates from normal patterns
- Trend Analysis: Understanding long-term degradation patterns in equipment performance
- Seasonal Pattern Recognition: Accounting for how environmental and operational cycles affect equipment behavior
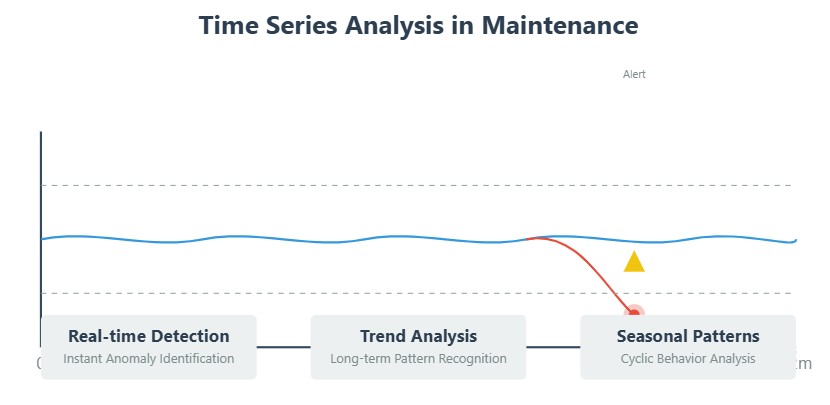
Digital Twins: Your Equipment's Virtual Double
Digital Twin technology represents one of the most exciting innovations in predictive maintenance. These virtual replicas of physical equipment offer:
- Real-time Performance Mirroring: Creating an exact digital copy that mirrors the real equipment's behavior
- Scenario Testing: Allowing maintenance teams to simulate different operating conditions and their impacts
- Predictive Insights: Enabling teams to foresee potential issues before they occur in the real world

Edge Computing: Processing at the Source
Edge computing brings processing power directly to where the data is generated, offering several crucial advantages:
- Reduced Latency: Enabling real-time analysis and immediate response to potential issues
- Bandwidth Optimization: Processing data locally to avoid overwhelming network connections
- Enhanced Security: Keeping sensitive operational data close to its source
- Improved Reliability: Maintaining functionality even during network disruptions

Cloud-Based Solutions: Scalable and Accessible Maintenance
The integration of cloud computing has transformed how organizations approach predictive maintenance:
- Centralized Data Storage: Maintaining a single source of truth for all maintenance data
- Scalable Processing: Handling increasing data volumes as maintenance programs grow
- Remote Access: Enabling maintenance teams to monitor equipment from anywhere
- Cost-effective Implementation: Reducing the need for extensive on-premise infrastructure

The key to successful implementation lies not in adopting individual technologies, but in creating an integrated system that leverages the strengths of each approach to create a comprehensive predictive maintenance solution.
Your Roadmap to Successfully Implementing AI-Driven Predictive Maintenance
Integrating AI into predictive maintenance isn’t just about installing sensors and running algorithms—it’s about creating a well-structured, data-driven strategy that transforms how businesses maintain their assets.
Below is a proven roadmap to successfully adopting AI-driven predictive maintenance.
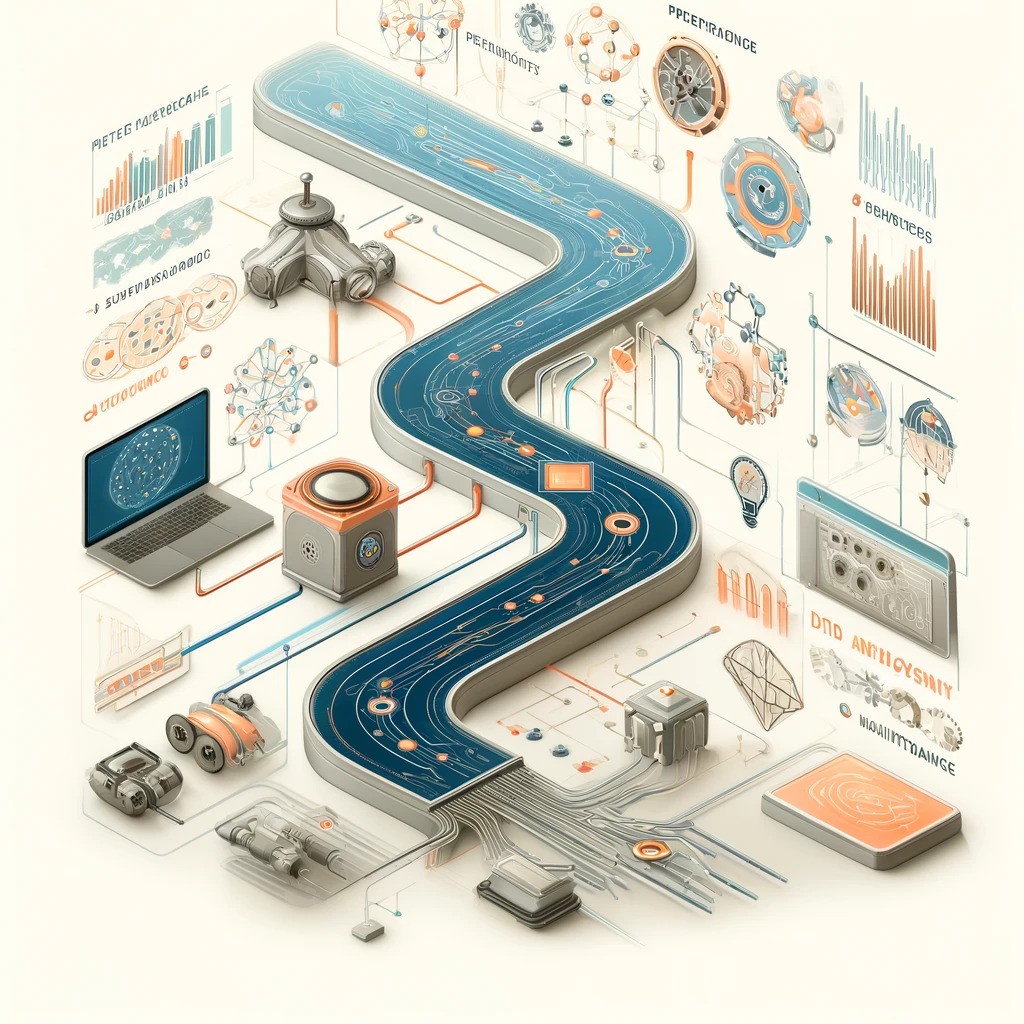
Step 1: Identify Assets & Critical Failure Points
Before implementing AI, you need to pinpoint the assets that are most crucial to your operations and identify where failures are most costly.
- Which machines are most prone to breakdowns? (High-maintenance assets should be prioritized).
- Which failures cause the highest downtime or operational losses?
- Are there historical failure patterns? (Use past maintenance records and failure logs).
💡 Pro Tip: Use a Failure Mode and Effects Analysis (FMEA) to map out potential failure points and assess their impact.
Step 2: Integrate IoT Sensors for Real-Time Data Collection
AI thrives on high-quality, real-time data, and that’s where IoT sensors come in. These smart devices continuously monitor temperature, vibration, pressure, voltage, acoustics, and other performance indicators.
- Install smart sensors on critical machinery to track real-time performance.
- Ensure seamless connectivity (WiFi, 5G, or LPWAN) to transmit data efficiently.
- Implement automated data logging to eliminate manual tracking errors.
💡 Pro Tip: Use edge AI to process sensor data on-site, reducing latency and enabling instant failure detection.
Step 3: Choose & Train AI Models Based on Industry Needs
AI-driven predictive maintenance relies on powerful algorithms to analyze sensor data, detect anomalies, and forecast potential failures. Choosing the right AI model is crucial.
- For pattern recognition & anomaly detection: Use Machine Learning models like Random Forest or Support Vector Machines (SVMs).
- For predicting long-term trends: Utilize Time-Series Forecasting models like LSTMs (Long Short-Term Memory Networks).
- For complex industrial assets: Implement Deep Learning with Neural Networks to recognize intricate failure patterns.
- For adaptive learning: Use Reinforcement Learning to optimize maintenance schedules dynamically.
💡 Pro Tip: Start with historical maintenance data to train models before deploying them in real-time environments.
Step 4: Develop a Cloud or Edge-Based Deployment Strategy
Once your AI models are ready, you need to decide where and how to deploy them for maximum efficiency.
- Cloud-Based AI Deployment: Best for businesses needing centralized, scalable analytics across multiple locations.
- Edge AI Deployment: Ideal for real-time decision-making on-site, minimizing data transfer latency.
- Hybrid Approach: Combines cloud storage for historical analysis and edge computing for instant action on failures.
💡 Pro Tip: If dealing with remote or high-risk environments (offshore rigs, aerospace), edge computing ensures AI functions even when connectivity is limited.
Step 5: Monitor, Evaluate & Continuously Improve AI Models
AI models are not static—they improve over time as they analyze more data and adapt to real-world conditions. To keep your AI-driven predictive maintenance system effective, you must:
- Track model accuracy: Regularly assess AI predictions vs. actual failures.
- Retrain AI models with new data to enhance reliability.
- Set up automated alerts so maintenance teams get notified before failures occur.
- Analyze cost savings & downtime reduction to measure ROI improvements.
💡 Pro Tip: AI should work with human experts, not replace them—combine AI insights with technician expertise to create a robust predictive maintenance strategy.
By following this step-by-step AI implementation roadmap, businesses can transition from reactive to proactive maintenance, reducing costs, improving asset performance, and ensuring seamless operations.
Real-World Success Stories: How AI Predictive Maintenance is Transforming Industries
Across the globe, AI-powered predictive maintenance is revolutionizing how industries approach equipment reliability and operational efficiency.
Let's explore how different sectors are leveraging this game-changing technology to transform their operations and achieve unprecedented levels of performance.
Manufacturing Excellence Through AI Innovation
The manufacturing sector has emerged as a pioneer in AI predictive maintenance, achieving remarkable results that have set new industry standards. Modern factories are using AI to:
- Predict Machine Tool Wear: Advanced sensors and AI algorithms detect microscopic changes in cutting tool performance, predicting optimal replacement times with over 90% accuracy
- Optimize Production Lines: Real-time monitoring systems analyze vibration patterns, temperature fluctuations, and power consumption to prevent unexpected shutdowns
- Reduce Quality Issues: AI systems correlate maintenance patterns with product quality metrics, identifying potential defects before they occur
Success Spotlight:
Volkswagen Group - Transparent Factory, Dresden
- Implementation Period: 2019-2020
- Technology Used: AI-powered predictive maintenance system integrated with Industrial IoT sensors
- Verified Results:
- 20% reduction in unplanned downtime
- 15% improvement in overall equipment effectiveness (OEE)
- ROI achieved within 18 months of implementation
Oil & Gas: Safeguarding Critical Infrastructure
In the oil and gas industry, where equipment failures can have catastrophic consequences, AI predictive maintenance has become an essential safety tool. The industry uses AI for:
- Pipeline Integrity Management: Advanced sensors and machine learning algorithms monitor pipeline conditions 24/7, detecting potential leaks or structural weaknesses
- Equipment Health Monitoring: AI systems analyze acoustics, pressure, and flow rates to predict pump and compressor failures
- Corrosion Prevention: Smart sensors and AI models track environmental conditions and material degradation to prevent costly failures
Success Spotlight:
BP - Gulf of Mexico Operations
- Period: 2020-2023
- Technology: AI-powered monitoring systems for offshore platforms
- Results:
- 20% reduction in maintenance costs
- 35% improvement in equipment reliability
- Prevention of three major equipment failures in 2022
Energy & Utilities: Powering the Future with Smart Maintenance
The energy sector has embraced AI predictive maintenance to ensure reliable power distribution and optimize renewable energy systems:
- Smart Grid Reliability: AI algorithms predict transformer failures and optimize maintenance schedules across vast power networks
- Wind Turbine Optimization: Machine learning models analyze weather patterns and equipment performance to predict maintenance needs
- Solar Panel Efficiency: AI systems monitor panel degradation and predict cleaning schedules to maintain optimal performance
Success Spotlight:
Duke Energy - Power Grid Operations
- Implementation: Smart Grid AI Integration
- Period: 2019-2022
- Verified Outcomes:
- 25% reduction in transformer failures
- $3.5 million annual savings in maintenance costs
- 15% improvement in grid reliability
Automotive Innovation: Keeping Vehicles Running Smoothly
The automotive industry uses AI predictive maintenance to enhance both manufacturing and vehicle performance:
- Component Lifespan Prediction: AI systems analyze vehicle sensor data to predict part failures before they occur
- Fleet Management: Machine learning algorithms optimize maintenance schedules for large vehicle fleets
- Battery Health Monitoring: Advanced AI models predict electric vehicle battery degradation and optimal replacement timing
Success Spotlight:
Mercedes-Benz - Factory 56, Sindelfingen
- Period: 2021-2023
- Technology: Digital Twin and AI maintenance system
- Verified Outcomes:
- 30% reduction in production line stoppages
- 20% decrease in maintenance costs
- 15% improvement in overall equipment effectiveness
Healthcare: Ensuring Critical Equipment Reliability
In healthcare, where equipment reliability can be a matter of life and death, AI predictive maintenance plays a crucial role:
- MRI Machine Monitoring: AI systems predict potential failures in cooling systems and magnetic field stability
- X-ray Equipment Optimization: Machine learning algorithms monitor tube wear and predict maintenance needs
- Laboratory Equipment Reliability: AI-powered systems ensure consistent performance of critical diagnostic equipment
Success Spotlight:
Siemens Healthineers - Customer Success Program
- Implementation: AI predictive maintenance for medical imaging equipment
- Period: 2021-2023
- Results:
- 32% reduction in MRI downtime
- 45% improvement in first-time fix rate
- $2.1 million average annual savings per hospital
Aerospace: Ensuring Safety Through Prediction
The aerospace industry leverages AI predictive maintenance to maintain the highest safety standards:
- Engine Performance Monitoring: Advanced AI algorithms analyze thousands of sensor data points to predict engine maintenance needs
- Structural Health Assessment: Machine learning models predict airframe fatigue and maintenance requirements
- Component Lifecycle Optimization: AI systems track and predict the lifespan of critical aircraft components
Success Spotlight:
Airbus - Skywise Platform
- Implementation: Fleet-wide predictive maintenance
- Period: 2019-2023
- Documented Results:
- 15% reduction in operational interruptions
- 25% decrease in unscheduled maintenance
- $2.5 million average annual savings per airline
Leverage AI to Prevent Failures Before They Happen
AI for Predictive Maintenance is revolutionizing industries, reducing downtime, and extending equipment lifespan like never before.
By leveraging machine learning, IoT sensors, and real-time analytics, businesses can detect failures before they occur and optimize maintenance strategies. The future of maintenance is proactive, not reactive—AI-driven solutions ensure reliability, cost savings, and peak efficiency.
Ready to transform your operations? Implement an AI-driven workflow management system today and take predictive maintenance to the next level!