Did you know that human biases in stakeholder management can lead to costly project delays of up to 20%? However, with AI transforming the way we approach stakeholder management, these challenges are rapidly being minimized.
Imagine using algorithms that analyze sentiments, predict reactions, and even pinpoint potential conflicts before they happen—welcome to the world of AI-powered stakeholder analysis.
This cutting-edge approach brings precision to understanding stakeholder dynamics, making it easier than ever to optimize project outcomes.
In this article, we will explore:
- How to transform stakeholder management using AI-driven insights
- Implementing AI stakeholder analysis for improved project outcomes
- Overcoming challenges in AI integration for stakeholder analysis
- Selecting the best stakeholder approach tailored to your project
Boost Project Performance: 4 Game-Changing Ways AI Transforms Stakeholder Management
In the dynamic world of project management, understanding and managing stakeholders is crucial for success. AI-powered stakeholder analysis is revolutionizing this aspect, offering tangible improvements in project outcomes.
Below, we will explore four game-changing ways this innovative approach is transforming stakeholder management and driving project success.
1. Rapid and Efficient Stakeholder Data Analysis
AI tools have dramatically enhanced the speed and efficiency of stakeholder data analysis. Traditional methods often involve time-consuming manual processes, but AI can process vast amounts of data in a fraction of the time.
Key AI Technologies:
- Natural Language Processing (NLP)
- Quickly sifts through emails, social media posts, and project documents
- Extracts relevant stakeholder information automatically
- Machine Learning Algorithms
- Identify patterns and relationships in stakeholder data
- Uncover insights that might be missed by human analysts
- Automated Data Collection and Integration
- Gathers data from multiple sources
- Provides a comprehensive view of stakeholders without manual effort
Benefit: This rapid analysis allows project managers to make informed decisions quickly, responding to stakeholder needs and concerns in near real-time.
2. Real-Time Stakeholder Sentiment Analysis
One of the most powerful features of predictive stakeholder intelligence is its ability to provide real-time insights into stakeholder sentiments.
Capabilities:
- Continuous monitoring of stakeholder communications across various channels
- Detection of sentiment shifts as they occur
- Interpretation of emotional tone in stakeholder interactions
- Visualization of stakeholder sentiment trends through real-time dashboards
Impact: By staying ahead of stakeholder concerns, project managers can maintain positive relationships and address issues promptly, significantly reducing the risk of project derailment due to stakeholder dissatisfaction.
3. Predicting Stakeholder Influence and Impact
AI's predictive capabilities are perhaps its most groundbreaking contribution to stakeholder analysis. By leveraging historical data and complex algorithms, AI can:
- Forecast stakeholder reactions to project decisions or changes
- Identify key influencers within stakeholder groups
- Predict potential alliances or conflicts among stakeholders
Strategic Advantage: This predictive power enables project managers to strategically plan their stakeholder engagement, focusing resources where they'll have the most significant impact and preemptively addressing potential challenges.
4. Reducing Risks Through Improved Stakeholder Management
AI-assisted stakeholder evaluation significantly reduces the risks associated with stakeholder mismanagement. Here are some concrete examples:
Early Warning System
Scenario: Large infrastructure project
- AI detected growing discontent among community stakeholders by analyzing social media sentiment
- Project managers organized community engagement sessions, addressing concerns proactively
- Result: Avoided a potential 6-month delay and $2 million in additional costs
Stakeholder Alignment
Scenario: Global software rollout
- AI analysis identified misaligned expectations among key stakeholders in different regions
- Project team tailored communication strategies for each region
- Outcome: Increased stakeholder satisfaction by 40% and improved adoption rates of the new software
Resource Allocation
Scenario: Product development project
- AI analysis identified an overlooked stakeholder group with significant influence
- Project team reallocated resources to engage this group effectively
- Result: Prevented late-stage design changes that would have cost an estimated $500,000
Conflict Prevention
Scenario: Merger between two companies
- AI predicted potential conflicts between stakeholder groups based on historical data
- HR teams implemented targeted team-building and culture alignment initiatives
- Outcome: Smooth integration process, with employee satisfaction 25% higher than industry average for similar mergers
This analysis represents a paradigm shift in how we understand and manage stakeholder relationships.
By providing faster analysis, real-time insights, predictive capabilities, and risk reduction, AI is enabling project managers to navigate the complex landscape of stakeholder management with unprecedented precision and effectiveness.
Master AI-Powered Stakeholder Analysis: Your All-in-One Implementation Roadmap
Integrating Intelligent stakeholder analysis into your project management processes can be a game-changer, but it requires careful planning and execution.
Here, you'll find a roadmap for successful implementation, covering integration steps, data best practices, and essential training requirements.
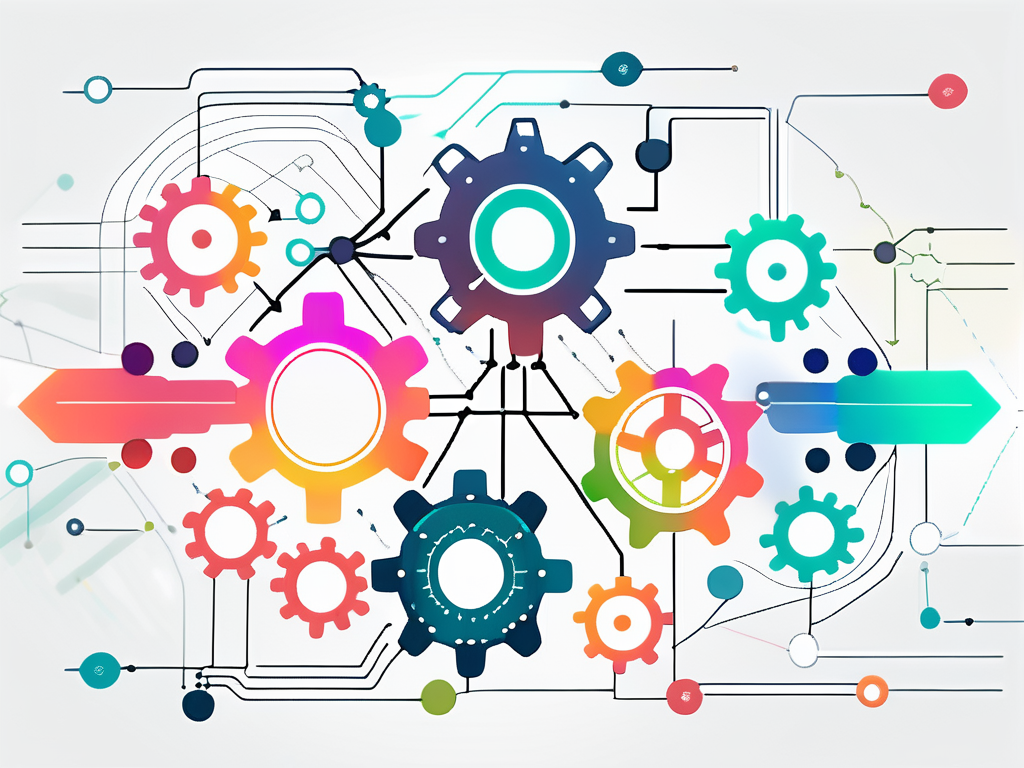
By following these strategies, you'll be well-equipped to harness the power of AI and dramatically improve your project outcomes and stakeholder relationships.
Step-by-Step Integration Guide
- Assess Your Current Stakeholder Management Process
- Identify pain points and areas for improvement
- Determine specific goals for AI implementation (e.g., faster analysis, better prediction)
- Choose the Right AI Solution
- Research available AI tools specifically designed for stakeholder analysis
- Consider factors such as compatibility with existing systems, scalability, and user-friendliness
💡 Tip: Look for solutions offering a free trial to test their effectiveness in your environment
- Prepare Your Infrastructure
- Ensure your IT infrastructure can support the chosen AI tool
- Address any data security concerns or compliance requirements
- Develop an Implementation Timeline
- Create a phased approach to minimize disruption
- Set realistic milestones for each stage of integration
- Pilot Implementation
- Start with a small-scale pilot project to test the AI tool
- Gather feedback from users and stakeholders
🔑 Key Action: Use insights from the pilot to refine your full-scale implementation plan
- Full-Scale Rollout
- Gradually expand the use of AI across more projects
- Continuously monitor performance and gather user feedback
- Integrate with Existing Processes
- Align AI-powered analysis with your current stakeholder engagement strategies
- Update project management methodologies to incorporate AI insights
Best Practices for Data Collection and Preparation
The success of data-driven stakeholder analysis hinges on the quality and quantity of data you feed into the system. Follow these best practices to ensure optimal results:
Important: The more diverse and high-quality your data sources, the more comprehensive and accurate your stakeholder analysis will be.
Training Requirements for Project Teams
Successful implementation of AI-backed stakeholder monitoring requires more than just installing new software. Proper training is crucial for your team to leverage the full potential of these advanced tools.
📚 AI Literacy Training
- Provide basic education on AI concepts and capabilities
- Help team members understand the potential and limitations of AI in stakeholder analysis
🛠️ Tool-Specific Training
- Offer hands-on training sessions for the chosen AI stakeholder analysis tool
- Cover both basic functionality and advanced features
🧠 Data Interpretation Workshops
- Train team members to interpret AI-generated insights accurately
- Develop skills in translating AI outputs into actionable stakeholder management strategies
🤝 Ethical AI Use
- Educate teams on the ethical considerations of using AI in stakeholder analysis
- Emphasize the importance of maintaining human judgment alongside AI insights
🔄 Continuous Learning Programs
- Implement ongoing training to keep up with AI advancements
- Best Practice: Encourage knowledge sharing among team members through regular "AI in Action" sessions
🔁 Change Management Training
- Prepare teams for the cultural shift that comes with AI adoption
- Provide strategies for managing resistance to change among stakeholders
By following this comprehensive implementation plan, adhering to data best practices, and investing in thorough team training, you'll be well-positioned to harness the power of AI for enhanced stakeholder analysis.
Remember, the key to success lies in viewing AI as a powerful tool to augment human expertise, not replace it. With the right approach, it can dramatically improve your project outcomes and stakeholder relationships.
Overcoming Challenges in AI-Powered Stakeholder Analysis
Predictive stakeholder intelligence offers powerful benefits but comes with its own set of challenges. Let's explore three key issues and how to address them effectively.

1. Addressing Data Privacy and Security Concerns
The cornerstone of effective smart stakeholder analytics is data, but with great data comes great responsibility. Protecting stakeholder information is not just a legal obligation; it's crucial for maintaining trust and credibility.
To address privacy and security concerns, organizations should focus on three key areas:
- Robust Data Protection: Implement advanced encryption techniques for data storage and transmission. Regularly update security protocols and conduct frequent audits to stay ahead of emerging threats.
- Regulatory Compliance: Stay informed about relevant laws like GDPR and CCPA. Develop clear data handling policies and implement data anonymization techniques where appropriate.
- Transparency: Clearly communicate your data usage policies to stakeholders. Provide options for data control and opt-out, and regularly report on your protection measures.
By prioritizing data privacy and security, you not only comply with regulations but also build a foundation of trust with your stakeholders, essential for the success of your AI initiatives.
2. Managing Stakeholder Resistance to AI Adoption
Introducing AI into stakeholder analysis processes can face resistance due to fears of job displacement, mistrust of technology, or concerns about losing the "human touch". Overcoming this resistance is crucial for successful implementation.
Here's a three-pronged approach to manage stakeholder resistance:
- Education and Awareness Conduct workshops to demystify AI and its role in stakeholder analysis. Share success stories and case studies that demonstrate AI's benefits. Address misconceptions about AI replacing human roles head-on.
- Inclusive Implementation Involve key stakeholders in the AI tool selection and implementation process. Gather and act on feedback throughout the adoption journey. Highlight how AI augments human capabilities rather than replacing them.
- Tangible Benefits Demonstration Start with pilot projects that showcase quick wins and measurable improvements. Regularly communicate how AI is enhancing decision-making and project outcomes. Provide hands-on opportunities for stakeholders to experience AI tools firsthand.
📢 Remember: Successful AI adoption is as much about people as it is about technology. By addressing concerns directly and showcasing benefits, you can transform skeptics into advocates.
3. Ensuring Ethical Use of AI in Stakeholder Management
As AI becomes more integral to stakeholder analysis, ensuring its ethical use is paramount. This involves addressing issues of bias, fairness, and transparency in AI-driven decisions.
Consider the following framework for ethical AI use:
- Mitigate Algorithmic Bias Regularly audit your AI models for potential biases in stakeholder analysis. Ensure diversity in the data used to train AI models and implement checks and balances to identify and correct biased outputs.
- Maintain Human Oversight Establish clear processes for human review of AI-generated insights. Train team members to critically evaluate AI recommendations and foster a culture where challenging AI outputs is welcomed and expected.
- Promote Transparency Develop explainable AI models that can articulate the reasoning behind their outputs. Clearly communicate to stakeholders when AI is being used in analysis and decision-making. Provide mechanisms for stakeholders to question or appeal AI-driven decisions.
- Establish Ethical Guidelines Create a clear set of ethical principles governing the use of AI in stakeholder management. Regularly review and update these guidelines as AI technology evolves. Ensure all team members are trained on and committed to these ethical standards.
By prioritizing ethical considerations, you not only protect your stakeholders but also build a reputation as a responsible and trustworthy organization in the age of AI. While challenges in implementing Innovative stakeholder insights are significant, they are not insurmountable.
Proactively addressing data privacy concerns, managing resistance to adoption, and ensuring ethical use, you can unlock the transformative potential of AI in your stakeholder management processes.
Comparative Analysis: AI vs. Traditional Stakeholder Management
As project complexity grows, choosing between traditional and AI-powered stakeholder management becomes crucial. This analysis compares both approaches, highlighting their strengths and ideal use cases to help project managers make informed decisions.
Scenarios Where Traditional Methods May Be Preferable
- Small-scale projects with limited stakeholders
- Highly sensitive or confidential projects where data sharing is restricted
- Culturally sensitive contexts valuing personal relationships
- Areas with limited technological infrastructure
- Early project stages or highly innovative projects with unpredictable stakeholder reactions
Benefits of a Hybrid Approach
Combining AI-powered and traditional methods can leverage the strengths of both approaches:
- Use AI for initial data gathering and analysis, followed by human interpretation
- Employ traditional methods for relationship building, while using AI for ongoing monitoring
- Utilize AI to identify potential issues, which are then addressed through traditional engagement strategies
This integrated approach can lead to more robust, nuanced, and effective stakeholder management, balancing the efficiency of AI with the nuanced understanding provided by human insight.
Lead Your Projects to Success with AI-Enhanced Stakeholder Analysis
AI-driven stakeholder assessment is transforming how projects are managed, offering faster, more accurate insights into stakeholder needs, sentiment, and influence.
By leveraging AI, project managers can respond to real-time data, predict challenges before they arise, and align resources where they’ll make the greatest impact. With AI’s predictive capabilities, you can reduce risks, improve stakeholder satisfaction, and drive better project outcomes.
Ready to elevate your project management process? Dart, the AI-powered project management software, equips you with the tools to enhance stakeholder analysis and streamline your project workflows.