Did you know that NASA once lost a $125 million satellite due to a project coordination error? When dependencies break down, things literally fall out of the sky. If you're wondering how AI can assist with tracking project dependencies, you're not alone.
Teams are turning to intelligent systems not just to manage chaos—but to predict, prioritize, and prevent it before it becomes a costly mistake.
In this article, we will delve into:
- Reimagine project success with AI-powered dependency tracking
- Choose smarter methods over outdated manual tracking
- Discover top AI tools to simplify project dependencies
- See how Tesla uses AI to manage complex project dependencies
Supercharge Your Projects: How AI Revolutionizes Dependency Tracking
Project dependencies can quickly become a tangled web of interconnections that derail timelines, blow budgets, and frustrate team members. But there's good news on the horizon. Artificial intelligence is revolutionizing how we identify, track, and manage dependencies across even the most complex projects.
Let's explore the game-changing ways AI is transforming dependency management.
Automated Dependency Identification and Mapping
Gone are the days of manually mapping project dependencies through tedious analysis and team meetings. AI systems can now automatically detect relationships between tasks, resources, and deliverables by analyzing project documentation, code repositories, task descriptions, and historical project data.
These intelligent systems can identify:
- Direct dependencies where one task explicitly requires another's completion
- Implicit dependencies that humans might overlook but could impact project flow
- Resource dependencies showing conflicts or bottlenecks before they become problems
The result? A comprehensive dependency map created in minutes rather than days, with significantly fewer human errors and overlooked connections.
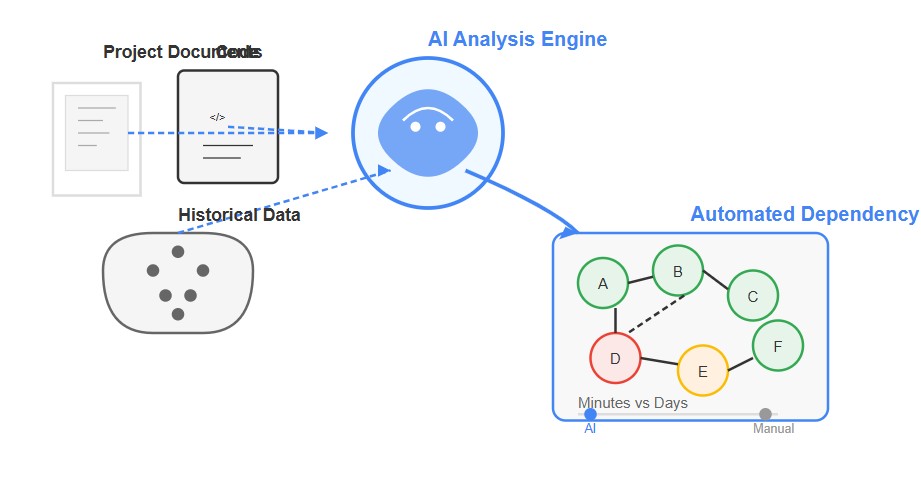
Real-Time Monitoring and Dynamic Updates
Project dependencies aren't static—they evolve as work progresses. AI provides continuous monitoring of your project landscape, instantly detecting when dependencies change due to:
- Scope modifications
- Timeline adjustments
- Resource availability shifts
- Requirement changes
When changes occur, AI systems automatically update dependency maps and alert stakeholders to potential impacts, eliminating the information lag that often leads to cascading delays. This real-time intelligence allows teams to maintain an accurate understanding of their project's interconnections at all times.

Predictive Analytics for Dependency-Related Risks
Perhaps AI's most valuable contribution is its ability to forecast potential dependency issues before they materialize. By analyzing patterns from thousands of similar projects and your own project history, AI can:
- Identify which dependencies carry the highest risk of delays
- Calculate the probability of dependency-related bottlenecks
- Estimate the potential impact of dependency failures
- Suggest proactive measures to mitigate risks
This predictive power shifts project management from reactive firefighting to strategic risk management, dramatically increasing delivery confidence.

Natural Language Processing for Documentation Analysis
Project dependencies often hide within volumes of documentation—requirements specifications, meeting notes, emails, and change requests. NLP (Natural Language Processing) technologies can extract dependency information from unstructured text, uncovering connections that might otherwise remain invisible.
AI systems can:
- Scan documentation to identify implied dependencies
- Flag inconsistencies in how dependencies are described
- Extract timeline dependencies from narrative descriptions
- Create structured dependency data from informal communications
This capability ensures that dependencies aren't missed simply because they weren't formally documented in project plans.
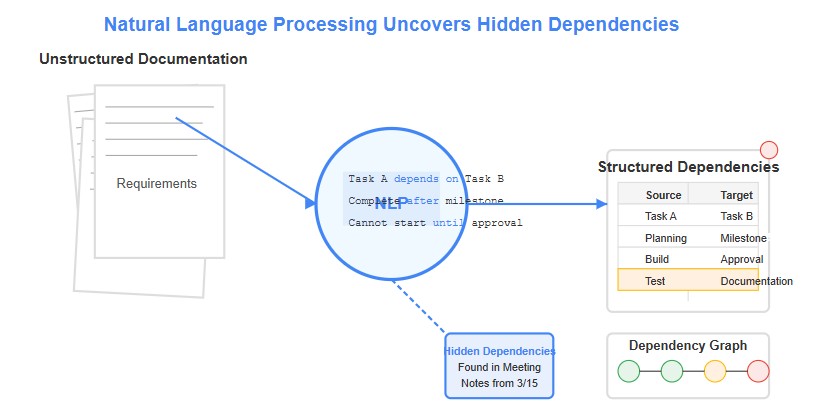
Machine Learning for Historical Pattern Recognition
Every organization builds a treasure trove of historical project data, but manually mining this information for dependency insights is practically impossible. Machine learning algorithms excel at discovering patterns in your project history that can inform current dependency management.
These systems can:
- Identify which types of dependencies historically cause the most delays
- Recognize patterns of successful dependency management
- Learn from past projects to improve dependency predictions
- Adapt to your organization's unique dependency challenges
Over time, these systems become increasingly accurate, learning from each project to better serve the next.

Visual Representation and Dependency Graphing
Complex dependency networks can be extraordinarily difficult to comprehend when presented as lists or tables. AI transforms dependency data into intuitive visual formats that make relationships immediately clear to all stakeholders.
Advanced visualization capabilities include:
- Interactive dependency graphs that respond to "what-if" scenarios
- Color-coded criticality indicators
- Dynamic visualizations that evolve as the project progresses
- Custom views tailored to different stakeholder concerns
These visual tools dramatically improve team communication around dependencies and ensure that complex relationship networks are understood by everyone from technical team members to executive sponsors.

Forward-thinking organizations are already using these intelligent solutions to predict bottlenecks, prevent cascading delays, and deliver projects with unprecedented reliability.
The question isn't whether you can afford to implement AI for dependency tracking—it's whether you can afford not to.
Make the Smart Shift: AI vs. Manual Dependency Management Compared
When managing complex projects, how you track dependencies can make or break your success. While traditional methods rely on manual analysis and static tools, AI brings speed, intelligence, and foresight to the process.
Let’s compare the two approaches so you can decide which one gives your team the edge.
Side-by-Side Comparison: AI vs. Manual Dependency Tracking
What Does This Mean for You?
While AI excels in speed, prediction, and scalability, it’s important to remember that human oversight is still essential—especially when interpreting context or making judgment calls. The real power comes when AI enhances your workflow rather than replacing it.
In short: AI doesn’t eliminate the need for smart project management—it amplifies it.
Power Up Your Project: 5 AI Tools That Transform Dependency Management
Stop drowning in dependency chaos and start leveraging artificial intelligence to automate, predict, and visualize your project connections.
These cutting-edge tools don't just track dependencies—they revolutionize how your team understands and manages them, helping you deliver complex projects with confidence and precision.
How to Choose the Right AI Dependency Tool for Your Team
With so many powerful tools available, the real challenge isn’t whether to adopt AI—it’s choosing the right platform that aligns with your team's unique needs. Here's how to make an informed decision:
- Understand your workflow complexity: If you're managing fast-moving agile projects with overlapping dependencies, tools like Dart or ClickUp AI offer the flexibility and visualization you need.
- Prioritize integrations: Ensure the AI tool connects with your current stack—Slack, GitHub, MS Teams, or Google Workspace—so you don’t have to reinvent your workflow.
- Consider your team's size and structure: Wrike is ideal for enterprise-scale operations, while Monday.com AI is great for mid-sized teams that value simplicity.
- Look beyond features—focus on outcomes: Choose a tool that doesn’t just track dependencies, but helps you predict risks, automate workflows, and deliver on time, every time.
The right AI tool isn’t just a software upgrade—it’s a strategic shift that transforms how your team thinks, plans, and delivers.
Case Study: How Tesla Uses AI to Anticipate and Manage Project Dependencies in Manufacturing
In large-scale manufacturing, project dependencies go beyond Gantt charts—they include machinery, workflows, timelines, and even ambient conditions.
Tesla, operating at the forefront of automation, faced an ongoing challenge: unexpected equipment failures were throwing entire production schedules off track, revealing hidden dependencies that traditional planning didn’t catch.
The Challenge: Hidden Dependencies Causing Downtime
At Tesla’s Gigafactories, highly specialized machines operate in sync to deliver just-in-time outputs. But when one critical machine failed, it triggered cascading delays across multiple production lines—a classic example of a missed dependency.
Despite scheduled maintenance plans, there was no way to predict which part of the system could become a bottleneck next.
The AI Solution: Predictive Dependency Mapping with Machine Learning
Tesla adopted an AI-driven predictive maintenance approach, not just to fix machines—but to map the operational dependencies between them. Using machine learning algorithms and real-time IoT sensor data (vibration, heat, acoustics), the system continuously:
- Monitored interdependencies between machines and stages in production
- Detected early warning signs of failure or performance degradation
- Mapped cause-effect chains of potential disruptions
- Alerted teams of which tasks or machines would be affected if a failure occurred
This gave Tesla visibility into how one issue could ripple through their workflow—a powerful form of dependency awareness.
The Outcome: More Predictable and Resilient Project Delivery
Implementing AI for predictive maintenance and dependency tracking led to:
- Proactive resource coordination to prevent cascading failures
- Reduced downtime with more accurate response prioritization
- Improved delivery timelines through early risk detection
- Smarter planning cycles thanks to insights from historical machine behavior
Lead With Clarity—Let AI Handle The Complexity
AI isn't just a tool—it’s your project team’s strategic advantage. From mapping tangled dependencies to predicting risks before they surface, AI transforms how you manage complexity.
Instead of reacting to problems, you’ll anticipate them. By adopting AI-driven solutions, you empower your team to move faster, collaborate better, and deliver with confidence. It’s time to leave guesswork behind and lead with clarity—because in today’s fast-paced project world, clarity is your most powerful edge.