Confusion over “who decides what” derails more projects than budget overruns or missed deadlines. In fact, unclear decision roles are a silent killer of team momentum. But what if you could fix that in minutes?
Learning how to generate a DACI framework with AI gives you the power to define accountability, streamline collaboration, and speed up decisions—without the politics, guesswork, or chaos.
In this article, we will delve into:
- Streamline decisions using these 5 AI-powered DACI steps
- Customize your DACI framework to fit any team structure
- Avoid critical DACI mistakes with help from AI
Transform Your Decision-Making: 5 Steps to Build Perfect DACI Frameworks with AI
In today's fast-paced business environment, clear decision-making processes are essential for organizational success. The DACI framework helps structure decisions and clarify roles, but creating them manually is time-consuming.
Enter artificial intelligence—your new decision framework architect that can revolutionize your process in these five actionable steps:
1. Gathering Project Requirements and Stakeholder Information
The foundation of any effective DACI framework is comprehensive information. Before engaging with AI tools:
- Document your project scope clearly, including objectives, timeline, and key deliverables
- Compile a complete list of all potential stakeholders across departments
- Identify existing organizational hierarchies and approval chains
- Outline the specific decisions that need to be made throughout the project lifecycle
Pro tip: Create a structured template for your project information that includes sections for goals, stakeholders, timeline, and decision points. This organized approach will significantly improve your AI outputs.
2. Selecting the Appropriate AI Tool for DACI Generation
Not all AI platforms are equally suited for generating decision frameworks. Consider these factors when selecting your AI assistant:
- Context understanding capabilities – Can the AI comprehend complex organizational structures?
- Project management knowledge – Does the AI understand DACI principles specifically?
- Customization options – Can you tailor the AI's outputs to match your organization's terminology?
- Integration capabilities – Will the AI output integrate with your existing project management tools?
Leading options include specialized project management AI tools like Asana's workflow assistant, as well as advanced language models that can be fine-tuned to understand organizational decision-making.
3. Prompting Techniques for Optimal DACI Framework Outputs
The quality of your DACI framework depends significantly on how you communicate with your AI tool. Effective prompting is an art that dramatically improves results:
- Begin with a clear definition of the DACI model to establish a baseline understanding
- Provide detailed context about your specific project and organization
- Specify any existing decision-making patterns your organization follows
- Include explicit requirements for the format and detail level of the output
- Ask the AI to justify its role assignments to ensure logical allocation
Example prompt structure:

4. Reviewing and Refining AI-Generated Frameworks
AI-generated DACI frameworks provide an excellent starting point, but human oversight remains crucial. When reviewing your AI output:
- Verify that all critical decisions have been captured
- Ensure role assignments align with organizational authority and expertise
- Check for balanced workload distribution among Drivers and Approvers
- Confirm that all necessary Contributors are included for specialized input
- Validate that the Informed list includes all affected stakeholders
Don't hesitate to iterate with your AI tool, providing feedback on what needs adjustment. Most advanced AI systems improve with specific guidance about what needs to be modified.
5. Implementing and Monitoring Your AI-Generated DACI Framework
The final step transitions from creation to action:
- Share the finalized framework with all stakeholders for transparency
- Integrate the DACI model into project management software where applicable
- Schedule regular check-ins to ensure the framework functions as intended
- Document any necessary adjustments for future AI-generated frameworks
Implementation is where many DACI frameworks fail, regardless of how they were created. Successful adoption requires deliberate change management and consistent reinforcement.
Real-World Example: AI-Generated DACI Framework for Product Launch
Let's examine how this process works in practice with a software company launching a new customer relationship management (CRM) feature.
Initial AI Prompt:
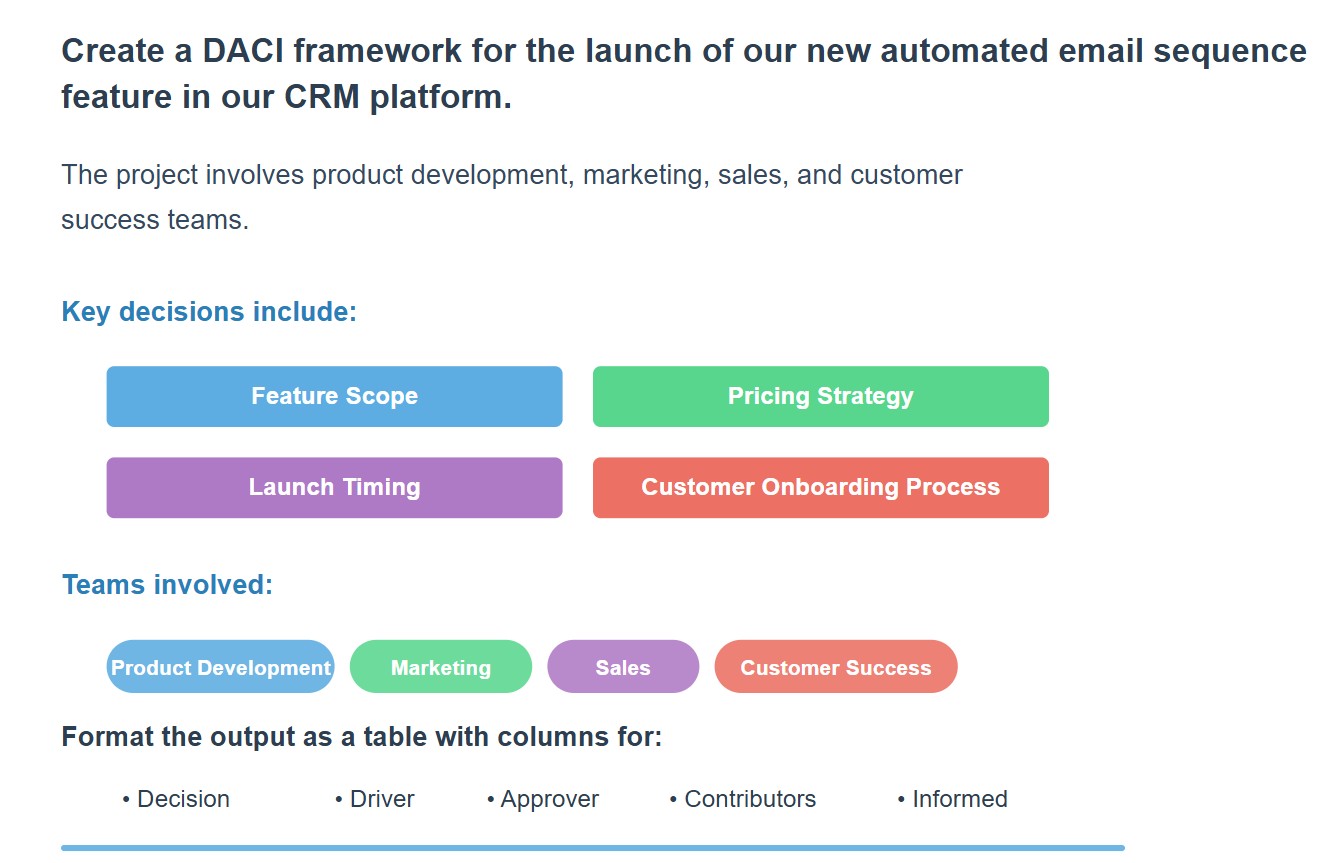
AI-Generated Framework (First Draft):
Human Review Identified Issues:
- The Customer Success team should contribute to pricing strategy decisions
- The CTO should be an Approver for launch timing due to technical dependencies
- The Content Marketing team was missing from Contributors for customer onboarding
After Refinement with AI:
The framework was updated with these adjustments, creating a more accurate decision structure that better reflected organizational expertise and authority.
When implemented, this AI-generated DACI framework helped reduce decision delays by approximately 25% and improved clarity in cross-departmental collaboration during the product launch process.
Team members reported a better understanding of their roles, and while not every deadline was met, the overall launch timeline saw fewer unexpected delays compared to previous projects.
The best DACI frameworks evolve with your projects. Use AI as an ongoing partner in refining your decision-making processes, not just as a one-time framework generator.
Tailor Your DACI Like a Pro: How to Customize AI-Generated Frameworks for Any Organization
AI can generate a solid DACI framework in seconds—but one size never fits all. Whether you're a lean startup, a global enterprise, or a distributed team spread across time zones, your organizational structure significantly impacts how decisions should be made.
Below, we show you how to adapt AI-generated DACI frameworks to match your team's unique dynamics, so they're not just efficient—they're effective.

Startup vs. Enterprise: Flexibility vs. Formality
The contrast between startup agility and enterprise structure demands fundamentally different approaches to decision frameworks, with AI capable of adapting to both extremes.
🟢 Startups
Startups are fast-moving and fluid. Roles often overlap, and decision-makers wear multiple hats. This requires a lean, adaptable DACI framework that reflects rapid iteration cycles.
How to customize with AI:
- Prompt AI to assume multi-role ownership (e.g., a Product Lead as both Driver and Contributor)
- Ask for simplified frameworks to avoid over-complication
- Emphasize speed over hierarchy in your AI prompts
Example: "Generate a DACI for a 5-person startup launching a new feature, where roles often overlap."
🔵 Enterprises
In large organizations, decisions pass through multiple layers of approval, and clear accountability is vital to avoid delays and confusion.
How to customize with AI:
- Ask AI to factor in hierarchies and compliance processes
- Include multiple contributors across regions or business units
- Reflect departmental specialization in role assignment
Tip: Use AI to generate a versioned DACI that evolves as decisions move up the chain.
Cross-Functional vs. Departmental Projects
The scope of your project—whether spanning multiple departments or contained within one—significantly affects how your DACI framework should be structured for optimal results.
🔀 Cross-Functional Projects
These involve diverse teams—marketing, product, engineering, sales—all with different perspectives.
How AI helps:
- Identifies natural contributors across departments
- Balances input while avoiding role overcrowding
- Ensures that Informed stakeholders aren't left behind
Prompt suggestion: "Generate a DACI for a product launch involving marketing, dev, support, and sales teams. Ensure balanced cross-department representation."
🧩 Departmental Projects
These are more siloed, often within a single function like IT or HR. They still benefit from DACI—but with tighter role clustering.
How to customize with AI:
- Ask for role depth within the same department
- Emphasize internal clarity over inter-team communication
- Keep frameworks concise to avoid redundancy
Example: In an HR policy rollout, HR might own all roles but distribute them clearly—Driver: HR Manager, Approver: HR Director, Contributors: HR Specialists, Informed: All Staff.
Adapting DACI Frameworks for Remote or Distributed Teams
Geographic dispersion creates unique decision-making challenges that require special consideration in your DACI structure to overcome distance barriers.
Remote work adds complexity to decision-making. Miscommunication, time zone gaps, and unclear ownership can quickly derail projects.
How AI can adapt your DACI for remote teams:
- Emphasize clarity and written documentation in role definitions
- Suggest asynchronous communication workflows
- Recommend splitting Driver/Contributor roles across time zones to maintain momentum
Prompt tip: "Create a DACI for a distributed team across three time zones working on a marketing campaign. Ensure async-friendly decision structure."
Bonus: AI can recommend tools (e.g., Notion, ClickUp, Loom) that support async DACI collaboration and updates.
AI-generated DACI frameworks are powerful—but not plug-and-play. To get real results, you must tailor the output to your team’s structure, workflow, and culture.
Fix It Before It Fails: 5 Costly DACI Mistakes You Can Avoid with AI
Even the most well-intentioned DACI frameworks can fall apart due to subtle human errors that impact project clarity and execution. These aren’t just small oversights—they’re critical breakdowns that lead to confusion, missed deadlines, and misaligned teams.
The good news? AI can help you catch and correct these issues—before they derail your projects.
Here are the five most common DACI framework mistakes and how AI can help you avoid them:
1. Proximity Bias: Choosing Familiar Faces Over Capable Contributors
It’s natural to assign roles to people we know and trust. But, when DACI frameworks are built based on personal familiarity instead of role relevance, it leads to misaligned accountability.
🛑 Mistake: A team lead assigns the Driver role to a colleague they frequently work with, even if that person isn't the most qualified.
🤖 How AI helps: AI evaluates project context and expertise objectively—suggesting Drivers and Contributors based on documented skill sets, project history, and decision proximity, not proximity to you.
AI helps surface hidden talent and silent contributors who often get overlooked.
2. Authority Bias: Equating Hierarchy with Decision-Making Power
Just because someone has a high-ranking title doesn’t mean they should be the Driver or Approver for every decision. Authority ≠ effectiveness.
🛑 Mistake: Assigning the CEO as Approver on every project milestone, leading to bottlenecks and slowdowns.
🤖 How AI helps: AI understands the actual decision-making needs of a task and suggests the right level of authority—not the highest title. It promotes smarter delegation and leaner workflows.
This enables faster decisions without sacrificing accountability.
3. Cross-Functional Blind Spots: Forgetting Critical Stakeholders
In complex projects, it’s easy to overlook secondary but essential departments. Ignoring them can cause miscommunication, resistance, or rework down the line.
🛑 Mistake: Leaving out the Customer Support team from a product launch DACI—only to find major usability issues after launch.
🤖 How AI helps: AI identifies cross-functional dependencies and recommends stakeholders based on role mapping, project type, and organizational patterns.
You get a well-rounded, inclusive framework that reduces risk and improves execution.
4. Workload Imbalance: Overburdening High-Performers
In many organizations, the same few people are always tagged as Drivers, Contributors, or Approvers. This leads to burnout and decision fatigue, even if those individuals are capable.
🛑 Mistake: Assigning the Product Manager to drive every aspect of the release plan.
🤖 How AI helps: AI detects over-assignment patterns and distributes responsibilities more equitably across the team. It can also suggest backup contributors or deputies.
This creates a more sustainable, collaborative decision-making environment.
5. Static Framework Syndrome: Failing to Adapt Over Time
Projects evolve. Yet many teams treat DACI frameworks as one-and-done documents. Without updates, they become irrelevant—and ignored.
🛑 Mistake: Using a static DACI created at project kickoff, even after scope or roles have shifted.
🤖 How AI helps: AI can suggest ongoing revisions to your DACI based on project progress, team changes, or added complexities. It keeps your framework live, relevant, and aligned.
Think of AI not just as a generator—but as a dynamic DACI co-pilot.
Empower Your Team by Defining Roles the Smart Way
Clear decision-making isn’t a luxury—it’s a project necessity. With the DACI framework and AI on your side, you can eliminate role confusion, align cross-functional teams, and accelerate execution without the drama.
Whether you're launching a product or rolling out a strategy, using AI to build your DACI means smarter starts and smoother finishes.
Ready to streamline decisions at scale? Try an AI-driven workflow management system tailored for structured team collaboration.