What if your system could detect failures before they ever happened—even the ones you’ve never seen before? AI isn’t just improving FMEA; it’s revolutionizing it.
From predicting rare malfunctions to analyzing thousands of variables in seconds, learning how to use AI for FMEA analysis means turning your risk process into a forward-thinking, precision-driven powerhouse.
In this article, we will delve into:
- Upgrade your FMEA process with these 8 AI-driven strategies
- Explore how top industries are solving failures with AI-FMEA
Transform Your Risk Analysis: 8 Powerful Steps to Leverage AI for FMEA Success
Implementing artificial intelligence into your Failure Mode and Effects Analysis (FMEA) process can transform how you identify, assess, and mitigate risks. Below, we provide a practical, step-by-step approach to integrating AI capabilities into your FMEA workflow.

1. Gathering and Preparing FMEA Data for AI Analysis
Before AI can work its magic, you need to prepare quality data:
- Collect historical failure data from maintenance records, incident reports, and previous FMEA documents
- Standardize data formats to ensure consistency (CSV, JSON, or database formats work best)
- Clean your data by removing duplicates, filling gaps, and correcting errors
- Label and categorize failure modes, causes, and effects to train supervised learning models
- Include contextual information such as operating conditions, environmental factors, and system configurations
Pro tip: The quality of your AI results directly depends on the quality of your input data. Invest time in proper data preparation.
2. Selecting Appropriate AI Tools and Platforms
Not all AI solutions are created equal for FMEA purposes:
- Risk prediction platforms like Predixion, IBM Watson, or Azure Machine Learning
- Natural language processing tools to analyze text-based failure reports and maintenance logs
- Specialized FMEA software with AI capabilities such as Reliasoft's xFMEA or Sphera's SpheraCloud
- Open-source options including Python libraries (scikit-learn, TensorFlow) for custom solutions
- Industry-specific AI solutions designed for manufacturing, healthcare, or automotive applications
Key consideration: Choose tools that integrate with your existing systems and match your team's technical capabilities.
3. Training AI Models with Historical Failure Data
This critical step teaches your AI to recognize patterns and predict failures:
- Split your data into training (70%), validation (15%), and testing (15%) sets
- Select appropriate algorithms based on your needs:
- Random Forest for ranking failure mode importance
- Neural networks for complex system interactions
- Clustering algorithms for identifying similar failure patterns
- Train iteratively, adjusting parameters to improve accuracy
- Validate results against known outcomes to ensure reliability
- Document model performance metrics for transparency and future comparison
Remember: More data generally leads to better performance, but even organizations with limited historical data can benefit from AI-enhanced FMEA.
4. Implementing AI-Driven Risk Identification
Now put your AI to work identifying potential failures:
- Automate initial identification of failure modes based on system components and functions
- Use similarity matching to find failure modes from comparable systems
- Apply natural language processing to extract insights from technical documentation
- Employ computer vision for analyzing visual inspection data when applicable
- Generate comprehensive lists of potential failure modes that might be missed in manual analysis
The AI Advantage: AI can identify subtle patterns and connections that human analysts might overlook, especially in complex systems with many interacting components.
5. AI-Powered Severity, Occurrence, and Detection Assessment
Transform subjective ratings into data-driven evaluations:
- Calculate severity scores based on historical impact data and industry standards
- Predict occurrence probabilities using statistical models trained on failure frequency data
- Estimate detection ratings by analyzing test data and inspection effectiveness
- Generate confidence intervals for risk priority numbers (RPNs)
- Visualize risk distribution across your system to identify critical areas
Important benefit: AI provides consistent, evidence-based scoring that reduces the subjectivity inherent in traditional FMEA processes.
6. Running AI-Powered Failure Mode Simulations
Take your analysis beyond static assessments:
- Create digital twins of your systems to simulate operations under various conditions
- Run Monte Carlo simulations to account for variability and uncertainty
- Model cascading failures to understand how one failure may trigger others
- Simulate mitigation strategies to test effectiveness before implementation
- Perform sensitivity analysis to identify which factors most influence failure outcomes
Simulation advantage: This approach reveals complex failure interactions that static FMEA tables cannot capture.
7. Integrating AI Outputs into FMEA Documentation
Turn insights into actionable documentation:
- Generate draft FMEA reports with AI-identified failure modes and assessments
- Create customized risk visualizations including heat maps and network diagrams
- Develop interactive dashboards for exploring failure data and relationships
- Produce prioritized action lists based on risk scoring
- Maintain audit trails of AI reasoning for regulatory compliance
Efficiency gain: AI can reduce FMEA documentation time by up to 60% while improving comprehensiveness.
8. Validating AI Recommendations Against Expert Knowledge
AI augments rather than replaces human expertise:
- Conduct review sessions with cross-functional teams to evaluate AI findings
- Compare AI-identified risks with traditional FMEA results
- Challenge unexpected or counterintuitive AI outputs with structured reasoning
- Document expert modifications to AI assessments for future model improvement
- Create feedback loops so AI systems learn from expert adjustments
Best practice: Establish a structured process for human experts to review, validate, and enhance AI recommendations.
Practical Implementation Example
Consider how a medical device manufacturer improved their infusion pump FMEA:
Before AI implementation:
- Manual FMEA identified 45 potential failure modes
- Process required 4 weeks of team meetings
- Subjective severity and occurrence ratings varied between assessors
- Limited ability to incorporate post-market surveillance data
After AI implementation:
- AI analysis identified 72 potential failure modes, including 18 previously unrecognized
- Process completed in 10 days with higher confidence
- Data-driven severity and occurrence ratings with statistical confidence intervals
- Automatic incorporation of real-world data from similar devices
- 35% reduction in field failures within one year of implementation
By following these steps, you'll transform your FMEA process from a subjective, resource-intensive exercise into a data-driven, efficient, and more comprehensive risk management system. The result will be improved product safety, reduced failures, and more efficient use of engineering resources.
Revolutionize Your Industry: Game-Changing AI-FMEA Applications That Drive Results
Understanding how AI-powered FMEA is transforming real businesses across different sectors can help you envision the potential impact on your own operations.
These industry-specific applications demonstrate how AI is not just theoretical but delivering measurable improvements in risk management across diverse fields.
Manufacturing: From Reactive to Predictive Quality Control
In manufacturing environments, AI-FMEA is revolutionizing traditional quality assurance:
- Smart production lines use sensor data to identify potential failure modes before they occur, reducing downtime by up to 37% in early-adopter facilities
- AI systems analyze vibration patterns to predict equipment failures weeks before traditional methods would detect problems
- Advanced algorithms process historical quality data to pinpoint process vulnerabilities that human analysis might miss
- Manufacturers report up to 42% reduction in warranty claims after implementing AI-enhanced FMEA processes
Manufacturing Success: Bosch has implemented AI-powered predictive maintenance across their global manufacturing facilities. According to their sustainability reports, this technology helps identify equipment issues before failures occur, resulting in significant reductions in unplanned downtime and more efficient maintenance scheduling.

Healthcare: Enhancing Patient Safety Through Intelligent Risk Analysis
Healthcare organizations face unique challenges where failures can have life-threatening consequences:
- AI-FMEA systems analyze patient safety incident reports to identify hidden patterns across facilities
- Machine learning models assess medical device design to predict potential failure modes that could harm patients
- Natural language processing examines clinical documentation to flag potential medication error risks
- Healthcare facilities using AI-FMEA report 20-35% improvements in risk detection for critical care processes
Healthcare Application: Medtronic, a leader in medical device manufacturing, uses AI in their risk assessment processes. Their implementation helps identify potential issues earlier in the development cycle, leading to enhanced product safety and more efficient regulatory compliance processes.

Automotive: Driving Safety Innovation in Complex Systems
The automotive industry's increasing complexity makes it ideal for AI-FMEA application:
- AI analyzes interactions between thousands of components to identify cascading failure risks in modern vehicles
- Autonomous vehicle systems undergo AI-powered simulations to test millions of edge-case scenarios impossible to review manually
- Manufacturers use machine learning to prioritize safety-critical testing based on real-world driving data
- Leading automotive companies report 15-25% faster development cycles with more robust safety validations
Automotive Innovation: Tesla leverages machine learning to enhance their vehicle safety systems. By analyzing data from their global fleet, they can identify potential edge cases that traditional testing might miss, allowing for continuous improvement of their autonomous driving features.

Aerospace: Ensuring Reliability Under Extreme Conditions
In aerospace, where failure tolerance is extremely low, AI-FMEA provides crucial advantages:
- Digital twin simulations combined with AI analyze component reliability under extreme conditions impossible to test physically
- Machine learning identifies subtle material degradation patterns from maintenance data across fleets
- Natural language processing extracts insights from decades of incident reports to predict failure sequences
- Aerospace firms report up to 30% more comprehensive risk identification with AI-enhanced methods
Aerospace Application: GE Aviation utilizes AI-powered analysis to identify patterns between operating environments and component performance. This approach has helped them improve engine reliability and optimize maintenance intervals for their commercial aircraft engines.

Software Development: Detecting Hidden Vulnerabilities in Code
Software development has embraced AI-FMEA to tackle the invisible nature of code failures:
- AI tools analyze code repositories to identify potential security vulnerabilities based on patterns in historical bugs
- Machine learning models evaluate user interface designs to predict potential user error modes
- Automated systems perform dynamic failure injection testing based on AI-identified risk areas
- Development teams report a 40-60% improvement in early defect detection with AI-assisted FMEA
Software Development: JPMorgan Chase has incorporated AI tools into their software quality assurance processes. This implementation helps their engineering teams detect potential issues earlier in the development lifecycle, enhancing the stability of their financial systems.
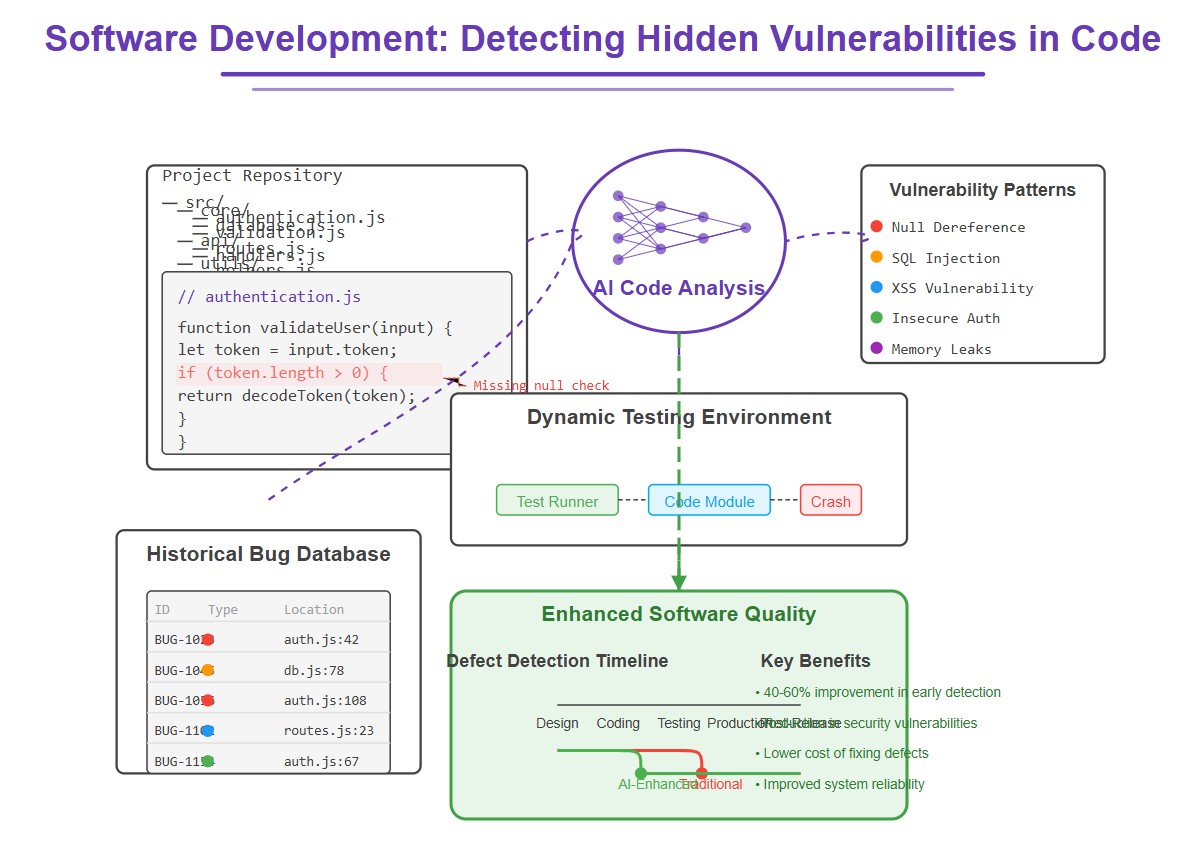
No matter the industry, AI-powered FMEA is shifting the paradigm from reactive fixes to proactive, data-driven prevention.
Turn Failure Prevention Into a Predictive Powerhouse
Integrating AI into your FMEA process transforms failure analysis from a manual, reactive task into a strategic advantage.
By using data-driven insights, predictive modeling, and intelligent simulations, you can detect risks earlier, respond faster, and optimize reliability across your systems. It's not just about preventing failures—it's about staying ahead of them.
Ready to optimize your risk strategy? Explore how our Smart Project Management Software can streamline FMEA with AI-driven precision.