Did you know that AI can process thousands of data points in mere seconds to forecast project delays and optimize resources?
While traditional methods often rely on intuition and past experiences, AI-powered predictive analytics takes things to a whole new level by analyzing patterns hidden deep within project data. In fact, some experts predict that AI will save companies up to 30% of their project costs in the next decade!
In this article, we will delve into:
- How to transform project management using AI-driven forecasting
- Optimizing AI forecasts with high-quality data inputs
- Insights from industry leaders using AI for project forecasting
- Tackling challenges in AI-powered project predictions
Revolutionizing Project Management: Harnessing the Power of AI-Driven Forecasting
In the ever-evolving landscape of project management, a groundbreaking technology is reshaping how we anticipate, plan, and execute projects.
AI-powered predictive analytics is not just a buzzword; it's a transformative force that's redefining the very essence of project forecasting.
Understanding AI-Powered Predictive Analytics in Project Management
At its core, AI-powered predictive analytics in project management refers to the application of advanced artificial intelligence and machine learning algorithms to analyze vast amounts of project data. This analysis goes far beyond simple number crunching. It involves:
- Pattern Recognition: Identifying subtle trends and correlations in historical project data that human analysts might overlook.
- Real-Time Processing: Continuously updating predictions as new data becomes available, ensuring forecasts remain current and relevant.
- Multi-Dimensional Analysis: Considering a wide array of factors simultaneously, from team performance metrics to external market conditions.
Key Benefits of AI-Driven Forecasting
The benefits of embracing AI-driven forecasting in project management are both numerous and significant:
- Enhanced Accuracy: AI algorithms can process and analyze data at a scale and speed impossible for human analysts, leading to more precise predictions of project outcomes, timelines, and resource needs.
- Proactive Risk Management: By identifying potential issues before they escalate, AI enables project managers to implement preventive measures, significantly reducing the impact of unforeseen challenges.
- Optimized Resource Allocation: AI can suggest the most efficient distribution of resources based on project needs, team skills, and availability, maximizing productivity and minimizing waste.
- Data-Driven Decision Making: With AI-generated insights, project managers can make informed decisions backed by solid data, reducing reliance on gut feelings or outdated information.
Transforming Traditional Project Forecasting Methods
The transformation of traditional project forecasting methods by AI is nothing short of revolutionary.
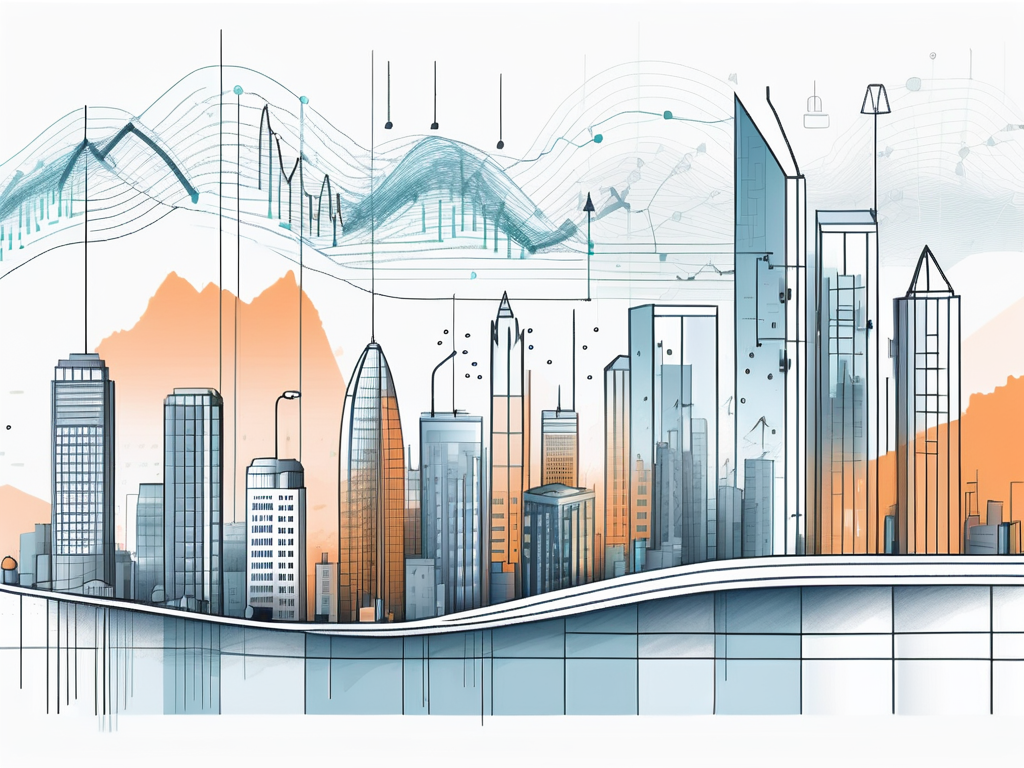
Where once project managers relied heavily on historical data and personal experience, AI brings a level of sophistication and adaptability previously unattainable. Here's how AI is changing the game:
- Dynamic Forecasting: Unlike static traditional methods, AI-powered forecasts adjust in real time as project conditions change, providing a constantly updated view of the project's trajectory.
- Scenario Analysis: AI can rapidly generate and evaluate multiple project scenarios, allowing managers to explore "what-if" situations and prepare for various outcomes.
- Automated Reporting: AI systems can generate comprehensive, easy-to-understand reports, freeing up valuable time for project managers to focus on strategic tasks.
- Predictive Maintenance: In projects involving equipment or infrastructure, AI can predict maintenance needs before breakdowns occur, minimizing downtime and costs.
By leveraging the power of AI, project managers are no longer limited by human cognitive constraints or processing power.
They can now tap into a wealth of insights that were previously inaccessible, leading to smarter planning, more efficient execution, and ultimately, higher rates of project success.
Data Requirements for Effective AI-Powered Project Forecasting
In the realm of AI-powered project forecasting, data is the lifeblood that fuels accurate predictions and insights. However, not all data is created equal.
To harness the full potential of AI in project management, it's crucial to understand the types of data needed, how to prepare it, and the ethical considerations surrounding its use.
The Data Goldmine: Types of Data for Accurate Predictions
AI thrives on diverse, comprehensive data sets. For project forecasting, the following types of data are essential:
- Historical Project Data: This forms the foundation of AI predictions. It includes:some text
- Past project timelines and milestones
- Budget information and cost breakdowns
- Resource allocation records
- Documented challenges and solutions
- Current Project Metrics: Real-time data from ongoing projects, such as:some text
- Task completion rates
- Resource utilization
- Budget expenditure
- Stakeholder feedback
- External Factors: Data that provides context to project conditions:some text
- Market trends and economic indicators
- Weather patterns (for construction or outdoor projects)
- Regulatory changes
- Competitor activities
- Team Performance Data: Insights into the human element of projects:some text
- Individual and team productivity metrics
- Skill sets and expertise levels
- Collaboration patterns
- Learning and development progress
By combining these data types, AI can create a holistic view of project dynamics, leading to more accurate forecasts and insights.
Garbage In, Garbage Out: Data Quality and Preparation Techniques
Even the most sophisticated AI algorithms can't compensate for poor-quality data. To ensure your AI-powered forecasting is built on a solid foundation, consider these data preparation techniques:
1. Data Cleaning
Start by removing duplicates, correcting errors, and standardizing formats. This process, while time-consuming, is crucial for accurate predictions.
2. Data Integration
Combine data from various sources into a unified dataset. This might involve merging databases, reconciling different naming conventions, and ensuring consistency across data points.
3. Feature Engineering
Create relevant features that AI models can use effectively. This might include deriving new metrics from existing data, such as calculating productivity rates or risk scores.
4. Data Validation
Implement rigorous checks to ensure data accuracy and relevance. This could involve cross-referencing data points, conducting statistical analyses, and having domain experts review the data.
5. Continuous Updates
Establish processes for regular data updates and maintenance. AI models perform best when they have access to the most current information.
Remember, the quality of your data directly impacts the accuracy of your AI forecasts. Investing time and resources in data preparation pays dividends in the form of more reliable predictions and insights.
Navigating the Ethical Maze: Considerations and Data Privacy
As we harness the power of data for AI-powered project forecasting, it's crucial to navigate the ethical considerations and privacy concerns that come with it.
Ethical Considerations
- Bias Prevention: Regularly audit your data and AI models for potential biases. Ensure your data represents diverse project scenarios and team compositions to avoid perpetuating existing biases.
- Transparency: Be open about how data is being used in your AI forecasting. Team members and stakeholders should understand what data is being collected and how it influences decisions.
- Human Oversight: While AI can provide powerful insights, it's essential to maintain human judgment in decision-making processes. Use AI as a tool to augment, not replace, human expertise.
Data Privacy
Protecting sensitive information is paramount. Consider these measures:
- Data Anonymization: Where possible, anonymize personal data to protect individual privacy.
- Access Controls: Implement strict access controls to ensure only authorized personnel can view sensitive project data.
- Compliance: Stay informed about and comply with data protection regulations such as GDPR, CCPA, or industry-specific guidelines.
- Consent and Transparency: Obtain necessary permissions for data utilization and be transparent about how the data will be used.
- Secure Storage and Transmission: Use encryption and other security measures to protect data both at rest and in transit.
By addressing these ethical and privacy concerns, you not only protect your organization and team members but also build trust in your AI-powered forecasting system.
Remember, the goal is not just to collect data, but to transform it into actionable insights that drive project success. With the right approach to data management, AI-powered project forecasting becomes not just a tool, but a strategic advantage in the complex world of project management.
Case Studies: Successful Implementation of AI Forecasting in Projects
The transformative power of AI-driven forecasting in project management is evident across various industries.
Let's explore how innovative companies have leveraged AI to revolutionize their project outcomes, and the valuable insights we can glean from their experiences.

1. Construction Industry: AECOM's AI-Powered Project Planning
Company: AECOM
Project: Predictive Analytics for Infrastructure Projects
AECOM, a global infrastructure consulting firm, implemented an AI-driven system for planning and managing large-scale infrastructure projects.
Implementation:
- Developed a machine learning model trained on historical project data
- Integrated real-time data from IoT sensors on construction sites
- Implemented AI-driven risk assessment and mitigation strategies
Quantitative Improvements:
- 22% reduction in project completion time
- 18% decrease in cost overruns
- 35% improvement in safety incident prediction and prevention
Lessons Learned:
- AI can effectively handle the complexities of large-scale infrastructure projects
- Real-time data integration significantly enhances forecast accuracy
- Predictive safety measures have both ethical and financial benefits
2. IT Industry: Atlassian's AI-Enhanced Project Management
Company: Atlassian
Project: Machine Learning Integration in Jira Software
Atlassian, known for its project management and collaboration tools, incorporated AI capabilities into its flagship product, Jira.
Implementation:
- Developed AI algorithms for intelligent issue assignment and sprint planning
- Implemented natural language processing for automated ticket categorization
- Created predictive analytics for project timeline and resource forecasting
Quantitative Improvements:
- 30% increase in team productivity
- 25% reduction in time spent on administrative tasks
- 20% improvement in sprint goal achievement rates
Lessons Learned:
- AI can significantly streamline routine project management tasks
- Machine learning models need continuous refinement based on user feedback
- Balancing automation with user control is crucial for widespread adoption
3. Manufacturing Industry: General Electric's Brilliant Factory
Company: General Electric (GE)
Project: AI-Driven Smart Manufacturing
GE implemented its "Brilliant Factory" concept, utilizing AI for optimizing manufacturing processes and project management.
Implementation:
- Developed digital twins of manufacturing processes for AI analysis
- Implemented machine learning for predictive maintenance and quality control
- Used AI for supply chain optimization and production scheduling
Quantitative Improvements:
- 20% increase in manufacturing productivity
- 30% reduction in maintenance costs
- 15% improvement in on-time delivery performance
Lessons Learned:
- Digital twin technology provides a powerful foundation for AI-driven optimization
- AI can effectively manage the complexities of global manufacturing networks
- Cross-functional collaboration is essential for successful AI implementation
4. Pharmaceutical Industry: Novartis's AI for Drug Development
Company: Novartis
Project: AI-Powered Clinical Trial Management
Novartis, a global healthcare company, implemented AI to optimize its clinical trial processes and project management.
Implementation:
- Developed AI models for patient recruitment and retention strategies
- Implemented machine learning for protocol optimization and site selection
- Used predictive analytics for resource allocation and timeline forecasting
Quantitative Improvements:
- 30% reduction in patient recruitment time
- 25% decrease in clinical trial costs
- 40% improvement in protocol design efficiency
Lessons Learned:
- AI can navigate the complexities of regulatory compliance in healthcare
- Ethical considerations are paramount when applying AI to human subjects research
- AI can significantly accelerate the drug development process
These case studies illustrate that AI-powered forecasting is a versatile tool driving innovation across sectors.
By adopting these best practices and learning from industry leaders, organizations can harness AI to transform their project management processes and achieve unprecedented levels of efficiency and success.
Navigating the Maze: Challenges and Limitations of AI-Powered Project Forecasting
While AI-powered project forecasting offers tremendous potential, it's not without its hurdles. Understanding these challenges is crucial for effective implementation and realistic expectations. Let's delve into the key limitations and how to address them.
The Shadow of Bias: Unmasking AI's Blind Spots
AI algorithms, despite their sophistication, can inadvertently perpetuate or even amplify biases present in their training data. This phenomenon, known as algorithmic bias, can lead to skewed project forecasts and potentially unfair decision-making.
Types of Bias in AI Forecasting:
- Historical Bias: When past project data reflects outdated practices or societal biases.
- Representation Bias: Occurs when certain groups or scenarios are under-represented in the training data.
- Measurement Bias: Arises from flawed data collection methods or metrics.
Mitigating Bias:
- Regular audits of AI models and their outputs
- Diverse and representative training data sets
- Cross-functional teams to review AI recommendations
- Transparency in AI decision-making processes
The Rearview Mirror Effect: Overreliance on Historical Data
AI models excel at identifying patterns in historical data, but this strength can become a limitation when facing unprecedented scenarios or rapidly changing environments.
Potential Pitfalls:
- Failure to anticipate novel risks or opportunities
- Outdated forecasts in dynamic markets or industries
- Reinforcement of past mistakes or inefficiencies
Strategies for Forward-Looking Forecasts:
- Incorporate real-time data and market trends
- Regularly update and retrain AI models
- Use scenario planning to prepare for various futures
- Combine AI insights with human expertise and intuition
The Human Touch: Balancing AI and Human Oversight
While AI can process vast amounts of data and identify complex patterns, it lacks human qualities like intuition, creativity, and ethical judgment. Effective project forecasting requires a delicate balance between AI capabilities and human oversight.
Areas Requiring Human Intervention:
- Contextual Understanding: Interpreting AI forecasts within broader business and societal contexts
- Ethical Considerations: Ensuring AI-driven decisions align with organizational values and societal norms
- Strategic Decision-Making: Using AI insights as inputs for high-level project strategy
- Stakeholder Communication: Translating AI forecasts into actionable insights for team members and clients
Best Practices for Human-AI Collaboration:
- Clear Roles and Responsibilities: Define where AI assists and where humans lead
- Continuous Learning: Train project managers in AI literacy and data interpretation
- Feedback Loops: Establish mechanisms for human feedback to improve AI models
- Ethical Guidelines: Develop and enforce clear ethical standards for AI use in project management
As we navigate the challenges of AI-powered project forecasting, it's crucial to remember that AI is a powerful tool, not a panacea. By acknowledging its limitations and thoughtfully integrating human oversight, we can harness the full potential of AI while mitigating its risks.
Transform Your Project Success Rate with AI Predictive Analytics
AI-powered predictive analytics is revolutionizing project forecasting, offering unprecedented accuracy, efficiency, and insight.
By harnessing real-time data, optimizing resources, and proactively identifying risks, AI empowers project managers to make smarter, data-driven decisions. From budget forecasting to timeline optimization, AI enhances every aspect of project management, transforming traditional methods into dynamic, future-ready strategies.
Ready to transform your projects? Explore how AI-powered tools can streamline workflows, optimize decision-making, and drive your success.